Sometimes debuggers and profilers are obviously broken, sometimes it's subtle and hard to spot. From my flame graphs page:
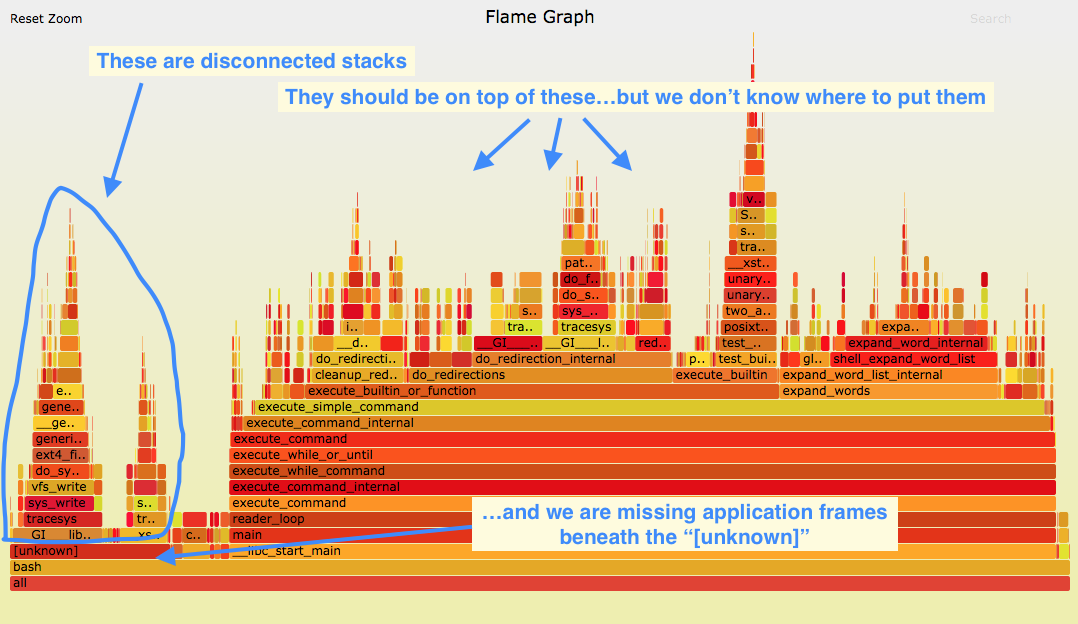
CPU flame graph (partly broken)
(Click for original SVG.) This is pretty common and usually goes unnoticed as the flame graph looks ok at first glance. But there are 15% of samples on the left, above "[unknown]", that are in the wrong place and missing frames. The problem is that this system has a default libc that has been compiled without frame pointers, so any stack walking stops at the libc layer, producing a partial stack that's missing the application frames. These partial stacks get grouped together on the left.
Other types of profiling hit this more often. Off-CPU flame graphs, for example, can be dominated by libc read/write and mutex functions, so without frame pointers end up mostly broken. Apart from library code, maybe your application doesn't have frame pointers either, in which case everything is broken.
I'm posting about this problem now because Fedora and Ubuntu are releasing versions that fix it, by compiling libc and more with frame pointers by default. This is great news as it not only fixes these flame graphs, but makes off-CPU flame graphs far more practical. This is also a win for continuous profilers (my employer, Intel, just announced one) as it makes customer adoption easier.
What are frame pointers?
The x86-64 ABI documentation shows how a CPU register, %rbp, can be used as a "base pointer" to a stack frame, aka the "frame pointer." I pictured how this is used to walk stack traces in my BPF book.
![]() Figure 3.3: Stack Frame with Base Pointer (x86-64 ABI) |
![]() Figure 2-6: Frame Pointer-based Stack Walking (BPF book) |
This stack-walking technique is commonly used by external profilers and debuggers, including Linux perf and eBPF, and ultimately visualized by flame graphs. However, the x86-64 ABI has a footnote [12] to say that this register use is optional:
"The conventional use of %rbp as a frame pointer for the stack frame may be avoided by using %rsp (the stack pointer) to index into the stack frame. This technique saves two instructions in the prologue and epilogue and makes one additional general-purpose register (%rbp) available."
(Trivia: I had penciled the frame pointer function prologue and epilogue on my Netflix office wall, lower left.)
2004: Their removal
In 2004 a compiler developer, Roger Sayle, changed gcc to stop generating frame pointers, writing:
"The simple patch below tweaks the i386 backend, such that we now default to the equivalent of "-fomit-frame-pointer -ffixed-ebp" on 32-bit targets"
i386 (32-bit microprocessors) only have four general purpose registers, so freeing up %ebp takes you from four to five (or if you include %si and %di, from six to seven). I'm sure this delivered large performance improvements and I wouldn't try arguing against it. Roger cited two other reasons for this change: The desire to outperform Intel's icc compiler, and the belief that it didn't break debuggers (of the time) since they supported other stack walking techniques.
2005-2023: The winter of broken profilers
However, the change was then applied to x86-64 (64-bit) as well, which had over a dozen registers and didn't benefit so much from freeing up one more. And there are debuggers/profilers that this change did break (typically system profilers, not language specific ones), more so today with eBPF, which didn't exist back then. As my former Sun Microsystems colleague Eric Schrock (nickname Schrock) wrote in November 2004:
"On i386, you at least had the advantage of increasing the number of usable registers by 20%. On amd64, adding a 17th general purpose register isn't going to open up a whole new world of compiler optimizations. You're just saving a pushl, movl, an series of operations that (for obvious reasons) is highly optimized on x86. And for leaf routines (which never establish a frame), this is a non-issue. Only in extreme circumstances does the cost (in processor time and I-cache footprint) translate to a tangible benefit - circumstances which usually resort to hand-coded assembly anyway. Given the benefit and the relative cost of losing debuggability, this hardly seems worth it."
In Schrock's conclusion:
"it's when people start compiling /usr/bin/ without frame pointers that it gets out of control."
This is exactly what happened on Linux, not just /usr/bin but also /usr/lib and application code! I'm sure there are people who are too new to the industry to remember the pre-2004 days when profilers would "just work" without OS and runtime changes.
2014: Java in Flames
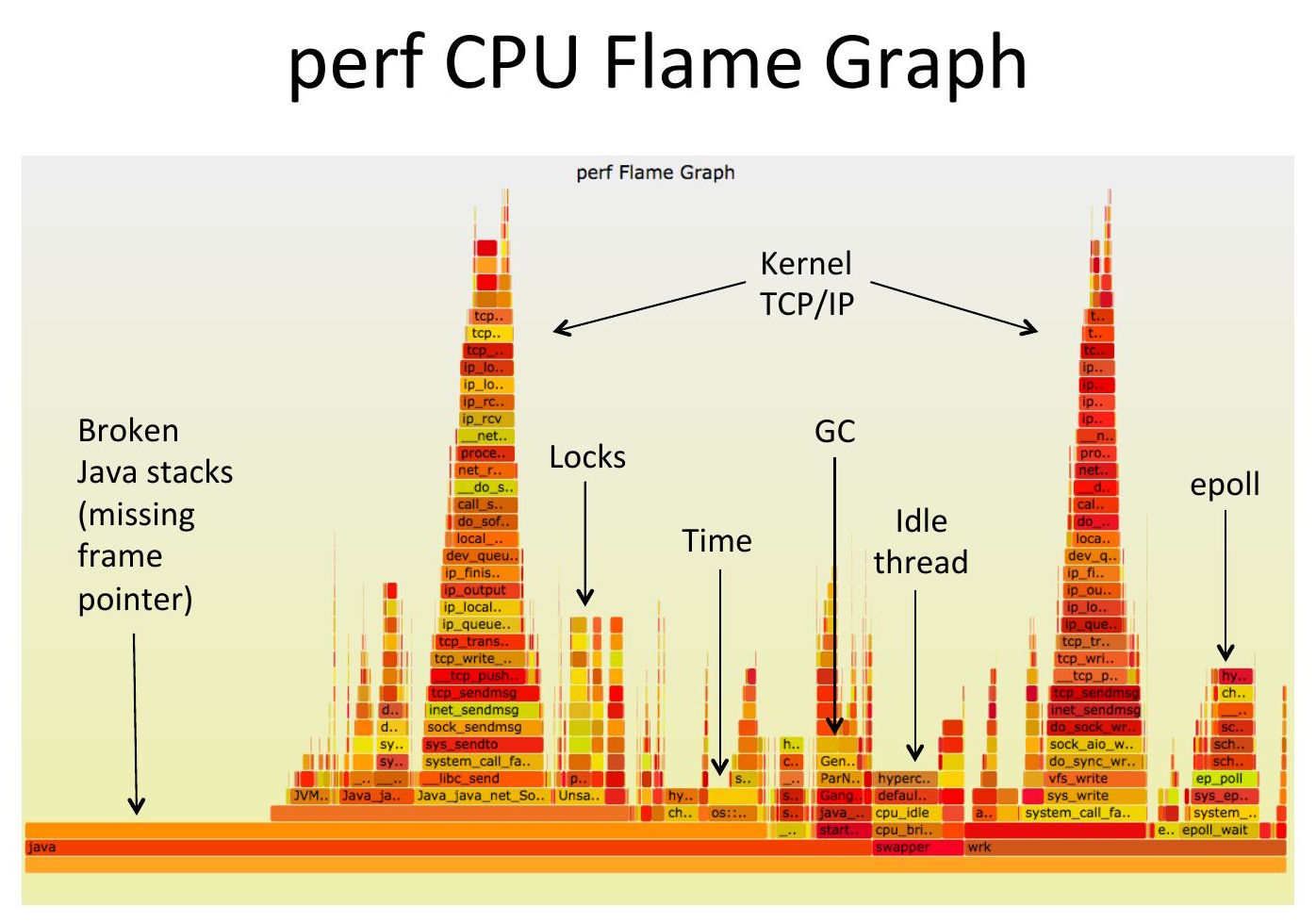
Broken Java Stacks (2014)
When I joined Netflix in 2014, I found Java's lack of frame pointer support broke all application stacks (pictured in my 2014 Surge talk on the right). I ended up developing a fix for the JVM c2 compiler which Oracle reworked and added as the -XX:+PreserveFramePointer option in JDK8u60 (see my Java in Flames post for details [PDF]).
While that Java change led to discovering countless performance wins in application code, libc was still breaking some portion of the samples (as pictured in the example at the top of this post) and was breaking most stacks in off-CPU flame graphs. I started by compiling my own libc for production use with frame pointers, and then worked with Canonical to have one prebuilt for Ubuntu. For a while I was promoting the use of Canonical's libc6-prof, which was libc6 with frame pointers.
2015-2020: Overhead
As part of production rollout I did many performance overhead tests, which I've described publicly before: The overhead of adding frame pointers to everything (libc and Java) was usually less than 1%, with one exception of 10%. That 10% was an unusual application that was generating stack traces over 1000 frames deep (via Groovy), so deep that it broke Linux's perf profiler. Arnaldo Carvalho de Melo (Red Hat) added the kernel.perf_event_max_stack sysctl just for this Netflix workload. It was also a virtual machine that lacked low-level hardware profiling capabilities, so I wasn't able to do cycle analysis to confirm that the 10% was entirely frame pointer-based.
The actual overhead depends on your workload. Others have reported around 1% and around 2%. Microbenchmarks can be the worst, hitting 10%: This doesn't surprise me since they resolve to running a small funciton in a loop, and adding any instructions to that function can cause it to spill out of L1 cache warmth (or cache lines) causing a drop in performance. If I were analyzing such a microbenchmark, apart from observability anaylsis (cycles, instructions, PMU, PMCs, PEBS) there is also an experiment I'd like to try:
- To test the theory of I-cache spillover: Compile the microbenchmark with and without frame pointers and find the performance delta. Then flame graph the microbenchmark to understand the hot function. Then add some inline assembly to the hot function where you add enough NOPs to the start and end to mimic the frame pointer prologue and epilogue (I recommend writing them on your office wall in pencil), compile it without frame pointers, disassemble the compiled binary to confirm those NOPs weren't stripped, and now test that. If the performance delta is still large (10%) you've confirmed that it is due to cache effects, and anyone who was worked at this level in production will tell you that it's the straw that broke the camel's back. Don't blame the straw, in this case, the frame pointers. Adding anything will cause the same effect. Having done this before, it reminds me of CSS programming: you make a little change here and everything breaks, and you spend hours chasing your own tail.
Another extreme example of overhead was the Python scimark_sparse_mat_mult benchmark, which could reach 10%. Fortunately this was analyzed by Andrii Nakryiko (Meta) who found it was a unusual case of a large function where gcc switched from %rsp offsets to %rbp-relative offsets, which took more bytes to store, causing performance issues. I've heard this has since been fixed so that Python can reenable frame pointers by default.
As I've seen frame pointers help find performance wins ranging from 5% to 500%, the typical "less than 1%" cost (or even 1% or 2% cost) is easily justified. But I'd rather the cost be zero, of course! We may get there with future technologies I'll cover later. In the meantime, frame pointers are the most practical way to find performance wins today.
What about Linux on devices where there is no chance of profiling or debugging, like electric toothbrushes? (I made that up, AFAIK they don't run Linux, but I may be wrong!) Sure, compile without frame pointers. The main users of this change are enterprise Linux. Back-end servers.
2022: Upstreaming, first attempt
Other large companies with OS and perf teams (Meta, Google) hinted strongly that they had already enabled frame pointers for everything years earlier. (Google should be no surprise because they pioneered continuous profiling.) So at this point you had Google, Meta, and Netflix running their own libc with frame pointers and able to enjoy profiling capabilities that most other companies – without dedicated OS teams – couldn't get working. Can't we just upstream this so everyone can benefit?
There's a bunch of difficulties when taking "works well for me" changes and trying to make them the default for everyone. Among the difficulties is that end-user companies don't have a clear return on the investment from telling their Linux vendor what they fixed, since they already fixed it. I guess the investment is quite small, we're talking about a single email, right?...Wrong! Your suggestion is now a 116-post thread where everyone is sharing different opinions and demanding this and that, as we found out the hard way. For Fedora, one person requested:
"Meta and/or Netflix should provide infrastructure for a side repository in which the change can be tested and benchmarked and the code size measured."
(Bear in mind that Netflix doesn't even use Fedora!)
Jonathan Corbet, who writes the best Linux articles, summarized this in "Fedora's tempest in a stack frame" which is so detailed that I feel PTSD when reading it. It's good that the Fedora community wants to be so careful, but I'd rather spend time discussing building something better than frame pointers, perhaps involving ORC, LBR, eBPF, and other technologies, than so much worry about looking bad in kitchen-sink benchmarks that I wouldn't trust in the first place.
2023, 2024: Frame Pointers in Fedora and Ubuntu!
Fedora revisited the proposal and has accepted it this time, making it the first distro to reenable frame pointers. Thank you!
Ubuntu has also announced frame pointers by default in Ubuntu 24.04 LTS. Thank you!
UPDATE: I've now heard that Arch Linux is also enabling frame pointers! Thanks Daan De Meyer (Meta).
While this fixes stack walking through OS libraries, you might find your application still doesn't support stack tracing, but that's typically much easier to fix. Java, for example, has the -XX:+PreserveFramePointer option. There were ways to get Golang to support frame pointers, but that became the default years ago. Just to name a couple of languages.
2034+: Beyond Frame Pointers
There's more than one way to walk a stack. These could be separate blog posts, but I want to comment briefly on alternates:
- LBR (Last Branch Record): Intel's hardware feature that was limited to 16 or 32 frames. Most application stacks are deeper, so this can't be used to build flame graphs, but it is better than nothing. I use it as a last resort as it gives me some stack insights.
- BTS (Branch Trace Store): Another Intel thing. Not so limited to stack depth, but has overhead from memory load/stores and BTS buffer overflow interrupt handling.
- AET (Archetectural Event Trace): Another Intel thing. It's a JTAG-based tracer that can trace low-level CPU, BIOS, and device events, and apparently can be used for stack traces as well. I haven't used it. (I spent years as a cloud customer where I couldn't access many HW-level things.) I hope it can be configured to output to main memory, and not just a physical debug port.
- DWARF: Binary debuginfo, has been used forever with debuggers. Update: I'd said it doesn't exist for JIT'd runtimes like the Java JVM, but others have pointed out there has been some JIT->DWARF work done. I still don't expect it to be practical on busy production servers that are constantly in c2. The overhead just to walk DWARF is also high, as it was designed for non-realtime use. Javier Honduvilla Coto (Polar Signals) did some interesting work using an eBPF walker to reduce the overhead, but...Java.
- eBPF stack walking: Mark Wielaard (Red Hat) demonstrated a Java JVM stack walker using SystemTap back at LinuxCon 2014, where an external tracer walked a runtime with no runtime support or help. Very cool. This can be done using eBPF as well. The performmance overhead could be too high, however, as it may mean a lot of user space reads of runtime internals depending on the runtime. It would also be brittle; such eBPF stack walkers should ship with the language code base and be maintained with it.
- ORC (oops rewind capability): The Linux kernel's new lightweight stack unwinder by Josh Poimboeuf (Red Hat) that has allowed newer kernels to remove frame pointers yet retain stack walking. You may be using ORC without realizing it; the rollout was smooth as the kernel profiler code was updated to support ORC (perf_callchain_kernel()->unwind_orc.c) at the same time as it was compiled to support ORC. Can't ORCs invade user space as well?
- SFrames (Stack Frames): ...which is what SFrames does: lightweight user stack unwinding based on ORC. There have been recent talks to explain them by Indu Bhagat (Oracle) and Steven Rostedt (Google). I should do a blog post just on SFrames.
- Shadow Stacks: A newer Intel and AMD security feature that can be configured to push function return addresses onto a separate HW stack so that they can be double checked when the return happens. Sounds like such a HW stack could also provide a stack trace, without frame pointers.
- (And this isn't even all of them.)
Daan De Meyer (Meta) did a nice summary as well of different stack walkers on the Fedora wiki.
So what's next? Here's my guesses:
- 2029: Ubuntu and Fedora release new versions with SFrames for OS components (including libc) and ditches frame pointers again. We'll have had five years of frame pointer-based performance wins and new innovations that make use of user space stacks (e.g., better automated bug reporting), and will hit the ground running with SFrames.
- 2034: Shadow stacks have been enabled by default for security, and then are used for all stack tracing.
Conclusion
I could say that times have changed and now the original 2004 reasons for omitting frame pointers are no longer valid in 2024. Those reasons were that it improved performance significantly on i386, that it didn't break the debuggers of the day (prior to eBPF), and that competing with another compiler (icc) was deemed important. Yes, times have indeed changed. But I should note that one engineer, Eric Schrock, claimed that it didn't make sense back in 2004 either when it was applied to x86-64, and I agree with him. Profiling has been broken for 20 years and we've only now just fixed it.
Fedora and Ubuntu have now returned frame pointers, which is great news. People should start running these releases in 2024 and will find that CPU flame graphs make more sense, Off-CPU flame graphs work for the first time, and other new things become possible. It's also a win for continuous profilers, as they don't need to convince their customers to make OS changes to get profiles to fully work.
Thanks
The online threads about this change aren't even everything, there have been many discussions, meetings, and work put into this, not just for frame pointers but other recent advances including ORC and SFrames. Special thanks to Andrii Nakryiko (Meta), Daan De Meyer (Meta), Davide Cavalca (Meta), Ian Rogers (Google), Steven Rostedt (Google), Josh Poimboeuf (Red Hat), Arjan Van De Ven (Intel), Indu Bhagat (Oracle), Mark Shuttleworth (Canonical), Jon Seager (Canonical), Oliver Smith (Canonical), Javier Honduvilla Coto (Polar Signals), Mark Wielaard (Red Hat), Ben Cotton (Red Hat), and many others (see the Fedora discussions). And thanks to Schrock.
Appendix: Fedora
For reference, here's my writeup for the Fedora change:
I enabled frame pointers at Netflix, for Java and glibc, and summarized the effect in BPF Performance Tools (page 40): "Last time I studied the performance gain from frame pointer omission in our production environment, it was usually less than one percent, and it was often so close to zero that it was difficult to measure. Many microservices at Netflix are running with the frame pointer reenabled, as the performance wins found by CPU profiling outweigh the tiny loss of performance." I've spent a lot of time analyzing frame pointer performance, and I did the original work to add them to the JVM (which became -XX:+PreserveFramePoiner). I was also working with another major Linux distro to make frame pointers the default in glibc, although I since changed jobs and that work has stalled. I'll pick it up again, but I'd be happy to see Fedora enable it in the meantime and be the first to do so. We need frame pointers enabled by default because of performance. Enterprise environments are monitored, continuously profiled, and analyzed on a regular basis, so this capability will indeed be put to use. It enables a world of debugging and new performance tools, and once you find a 500% perf win you have a different perspective about the <1% cost. Off-CPU flame graphs in particular need to walk the pthread functions in glibc as most blocking paths go through them; CPU flame graphs need them as well to reconnect the floating glibc tower of futex/pthread functions with the developers code frames. I see the comments about benchmark results of up to 10% slowdowns. It's good to look out for regressions, although in my experience all benchmarks are wrong or deeply misleading. You'll need to do cycle analysis (PEBS-based) to see where the extra cycles are, and if that makes any sense. Benchmarks can be super sensitive to degrading a single hot function (like "CPU benchmarks" that really just hammer one function in a loop), and if extra instructions (function prologue) bump it over a cache line or beyond L1 cache-warmth, then you can get a noticeable hit. This will happen to the next developer who adds code anyway (assuming such a hot function is real world) so the code change gets unfairly blamed. It will only regress in this particular scenario, and regression is inevitable. Hence why you need the cycle analysis ("active benchmarking") to make sense of this. There was one microservice that was an outlier and had a 10% performance loss with Java frame pointers enabled (not glibc, I've never seen a big loss there). 10% is huge. This was before PMCs were available in the cloud, so I could do little to debug it. Initially the microservice ran a "flame graph canary" instance with FPs for flame graphs, but the developers eventually just enabled FPs across the whole microservice as the gains they were finding outweighed the 10% cost. This was the only noticeable (as in, >1%) production regression we saw, and it was a microservice that was bonkers for a variety of reasons, including stack traces that were over 1000 frames deep (and that was after inlining! Over 3000 deep without. ACME added the perf_event_max_stack sysctl just so Netflix could profile this microservice, as the prior limit was 128). So one possibility is that the extra function prologue instructions add up if you frequently walk 1000 frames of stack (although I still don't entirely buy it). Another attribute was that the microservice had over 1 Gbyte of instruction text (!), and we may have been flying close to the edge of hardware cache warmth, where adding a bit more instructions caused a big drop. Both scenarios are debuggable with PMCs/PEBS, but we had none at the time. So while I think we need to debug those rare 10%s, we should also bear in mind that customers can recompile without FPs to get that performance back. (Although for that microservice, the developers chose to eat the 10% because it was so valuable!) I think frame pointers should be the default for enterprise OSes, and to opt out if/when necessary, and not the other way around. It's possible that some math functions in glibc should opt out of frame pointers (possibly fixing scimark, FWIW), but the rest (especially pthread) needs them. In the distant future, all runtimes should come with an eBPF stack walker, and the kernel should support hopping between FPs, ORC, LBR, and eBPF stack walking as necessary. We may reach a point where we can turn off FPs again. Or maybe that work will never get done. Turning on FPs now is an improvement we can do, and then we can improve it more later. For some more background: Eric Schrock (my former colleague at Sun Microsystems) described the then-recent gcc change in 2004 as "a dubious optimization that severely hinders debuggability" and that "it's when people start compiling /usr/bin/* without frame pointers that it gets out of control" I recommend reading his post: [0]. The original omit FP change was done for i386 that only had four general-purpose registers and saw big gains freeing up a fifth, and it assumed stack walking was a solved problem thanks to gdb(1) without considering real-time tracers, and the original change cites the need to compete with icc [1]. We have a different circumstance today -- 18 years later -- and it's time we updated this change. [0] http://web.archive.org/web/20131215093042/https://blogs.oracle.com/eschrock/entry/debugging_on_amd64_part_one [1] https://gcc.gnu.org/ml/gcc-patches/2004-08/msg01033.html